Indaba African Women in Machine Learning Evening
Kindly hosted by:
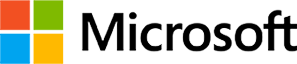
Registration/Invitation required.
When: Monday 26 August 2019 19:00-22:00
Venue: Kenyatta University Conference Centre (KUCC), Nairobi, Kenya
Our annual event dedicated to the African Women in Machine Learning.
Join our fantastic speakers at an event to encourage, support and unite women in machine learning, while highlighting diverse career paths: from academia, to industrial research, to applied machine learning, and start-ups. Our panellists will each describe their personal career journey, and their experiences as a woman in machine learning, followed by a panel discussion, Q&A from the audience and a chance to network. Free and open to all conference attendees.
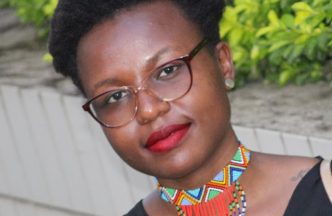
Kathleen Siminyu
Data Scientist Africa's Talking; Co-organiser, Nairobi Machine Learning and Data Science
Kathleen is a data scientist and machine learning engineer who is Regional Coordinator for the Artificial Intelligence for Development – Africa Network. She is Co-Founder and Co-Organiser of the Nairobi Women in Machine Learning and Data Science community as well as part of the Deep Learning Indaba leadership.
Kathleen is also currently a Masters student at the Georgia Institute of Technology undertaking the Online Masters in Computer Science with a specialization in Computational Perception and Robotics. She is keen on investing time and effort in ventures that involve natural language processing for African languages as well as low-cost hardware robotics.
She can be reached on twitter @siminyu_kat and on LinkedIn.
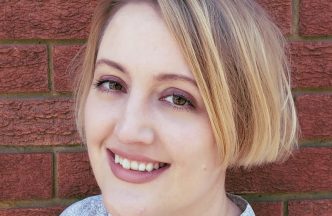
Tempest van Schaik
Microsoft Commercial Software Engineering
Tempest van Schaik is a multi-disciplinary engineer with experience in the end-to-end development of health technology, from wet-lab research, to medical devices, to clinical UX, and medical data science. She currently works as a machine learning engineer at Microsoft, London, focusing on healthcare & biosciences. She puts machine learning into practice in close collaboration with clinical & pharmaceutical researchers to solve diverse, real-world problems. Recently she has used ML to better understand the physiotherapy of Cystic Fibrosis. She has degrees in Biomedical Engineering, and Electrical (Information) Engineering from University of the Witwatersrand (South Africa), and a PhD in Bioengineering from Imperial College London. She is an Ambassador for Diversity & Inclusion for her team at Microsoft (Commercial Software Engineering).
You can find her on Twitter: @Dr_Tempest
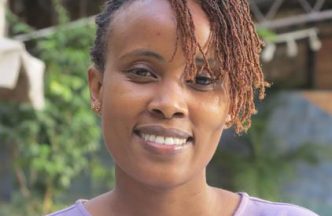
Grace Mutung’u
KICTANet
Grace Mutung’u researches ICT policy in Kenya and Africa, with a specialisation in digital rights, governance and development. She is a curator on Sub-Saharan Africa internet governance issues at the Geneva Internet Platform and an advocate of the High Court of Kenya. She is currently a visiting research fellow at the Centre for Intellectual Property and Information Technology (CIPIT) at Strathmore University, Kenya. She has been involved in ICT policy development over the past 10 years.
You can find her on Twitter @Bomu
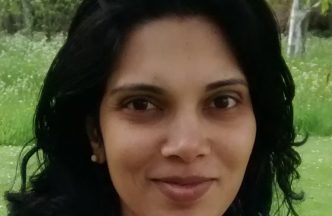
Pashmina Cameron
Microsoft Research, Cambridge UK
Pashmina leads the deep learning engineering team in All Data AI group of Microsoft Research, Cambridge. Since joining MSR in 2018, she has worked in various areas including healthcare, storage solutions and NLP. She has been part of the team responsible for Microsoft’s AI residency. This year-long program is designed to tackle the shortage of machine learning talent and combines research and engineering mentorship with working on real world solutions.
Before joining MSR, she led Computer vision R&D at Autonomy (HPE) and was responsible for IDOL MediaServer, a suite of computer vision solutions including face recognition, object recognition and intelligent scene analysis. Her research there spanned areas such as SLAM, 3D reconstruction, 3D change detection and augmented reality.
Prior to that, Pashmina won a Gates Cambridge scholarship to study for a PhD in Computer vision at the University of Cambridge. Her thesis explored novel feature extraction methods and evaluation strategies based on multi-view geometry.