By Amelia Taylor
Machine Learning (ML) is the talk of the tech-town. It is a tool everyone can use and it holds many promises: from being able to reveal hidden information in large data to being able to predict the “future” based on past data and sophisticated heuristics. ML is seen as the swiss army knife of big data and technology today. ML methods can be incorporated in almost everything: robotics, communication, manufacturing, medicine, finance, education, government, environment and so on.
ML is Global
Another important and attractive promise of ML is that it is available globally – it is within the reach of not only developed countries but also poorer countries, especially poorer countries! The annual pan-African events called Deep Learning Indaba have been gathering ML enthusiasts from Africa since 2018. DLI was started by Africa-born technologists motivated to apply ML to deep problems faced in Africa. DLI attracts hundreds of applications and are large (around 200 to 400 people!) These come from African IndabaX chapters but not only. IndabaX events are local ML-communities (now numbering 36). Here is a short write-up about the IndabaX-meter useful to read if you are looking for a ML-community in your own country.
From 2022, DLI has featured a session dedicated to African Research in ML, called Africa Research Showcase. This is in addition to the poster session with examples of applications of ML in African contexts. There are also the annual prizes for research and innovation in AI such as the Kambule Doctoral Award or the Maathai Impact Award. Clearly we all get excited about the impact that ML can make in these countries and we measure their ML-pulse at such events. What is interesting is that some countries (for example South Africa, Nigeria, Uganda, Tunisia) contribute with a higher number of posters and presentations than others. There are many reasons why this happens. Judging by the conversations taking place at the Deep Learning Indaba and IndabaX events we all want to find some answers. We need to develop some kind of diagnostic tool that measures ML-readiness – we expect that countries with a healthier ML-pulse score higher on our ML-readiness level. This will help us channel energy in boosting the ML research across all countries in Africa via the DLI/IndabaX networks.
I have not yet come across a ML-readiness tool – something similar to say the Technology Readiness Levels. This is a first humble attempt to come up with a tool to measure ML-readiness level.
Looking at the trends over the last few years we see that the ML market in Africa grew between 2013 and 2020 but has plateaued after that. The market is probably specialising but that we cannot know that from these numbers. It can also be that the market is not yet AI- ready or ML-ready.
ML Readiness – components
If you are an individual or organisation that wants to employ ML what do you need? I will venture to propose five things one needs. We will test their relevance in a series of blogs. These five components are:
- Large and reliable storage facility: to collect, store and annotate data.
- Processing power: fast and custom hardware capable to run and manage ML applications at great speed
- Integration capabilities to integrate ML applications in other components of an organisation or system.
- Reliable and Scalable ML code: Either being able to pay for ML libraries, or being able to use open-source libraries in a reliable and scalable way.
- Capital Investment and Risk Management
For the purpose of this discussion, ML-actors can be split into the following:
- Academic researchers such as students and lecturers who use ML on a small scale;
- Organisations (whether these are start-ups, universities, industry, government) seeking to use ML in their operations and processes.
We would like to start by looking at the grassroots levels – at individuals who work and develop applications using ML like the IndabaX or DLI participants. These smaller ML-actors are important because their work leads to experimental outputs which can later on be integrated by organisations – the second category of ML-actors.
The grassroots ML actors tend to do a combination of the following:
- use their own computers for running experiments.
- may be part of a network/ lab environment with sufficient means to produce technology demonstrations.
- rely on external expertise to run and develop algorithms (say an external collaboration with a better developed institution)
- use free or paid (many having a highly controlled budget) cloud services.
- have little funding available
- use open source ML-libraries.
- use open source datasets.
What do you do? Tell us by using this survey or emailing me at amelia@deeplearningindaba.com.
ML Readiness – dimensions
We can derive the following five dimensions which can provide indicators for our ML-readiness levels.
- Technology and skill base: access to good hardware for storage and computation; access to the internet; access to reliable electricity; access to people with good ML skills.
- Design: access to reliable ML-libraries, access to good quality and relevant datasets.
- Cost and Funding: access to research funding (if you are a university lecturer or student) or access to start-up funding.
- Process Capability and Control: being able to develop working prototypes, having a mature and replicable processes to scale and bring the ML-applications to the local market.
- Quality Management: using quality tools, being able to test the ML solutions and demonstrate their reliability and accuracy.
Neither of these are easy to measure exactly. We need to use proxies. Let us start with possible ways of measuring IndabaX countries along the first dimension: technology and skills base.
Proxies for measuring skill base can be:
- number of degrees with modules in ML or technological institutions (offering computer science and/or modules in ML)
- number of educational/training programs / organisations / labs / symposiums (IndabaX being one of them)
- number of research papers published / number of active research projects
- number of startups in ML
Proxies for measuring the technology base can be for example:
- access to internet (optical cable)/ internet penetration rate / cost of internet
- cost / access to required hardware/ cloud solutions
South Africa has been leading on the continent in terms of AI research centers and AI Startups. Over the last ten years, we saw the establishment of AI centers in other African countries: for example, Data Science Africa, Deep Learning Indaba, Google AI Lab Ghana, IBM research lab in Kenya, ICLR Conference in Ethiopia.
I think looking at countries that lead in technological and skills base measures is easier. It is harder to compare countries that are emerging as contestants such as Cameroon, Rwanda, Zambia. A good ML-readiness index should be able to pick up their visible progress.
Technology and skill base
Internet penetration: Top and bottom 5 IndabaX countries (Source Statistica). This refers to both mobile and optical fiber internet.
IndabaX Country (Top 5) | Internet penetration rate |
MOROCCO | 84% |
EGYPT | 72% |
SOUTH AFRICA | 68% |
TUNISIA | 67% |
ALGERIA | 61% |
IndabaX Country (Bottom 5) | Internet penetration rate |
MADAGASCAR | 22% |
MALAWI | 20% |
DR CONGO | 18% |
BURUNDI | 15% |
SOMALIA | 14% |
According to the African e-Connectivity Index 2021, South Africa is the top-ranked African country in terms of the quality of its internet connectivity with a score of 100 points, followed by Mauritius (96.56 points), Egypt (95.42), Kenya (89.60) and Tunisia (88.60). Nigeria ranks tenth.
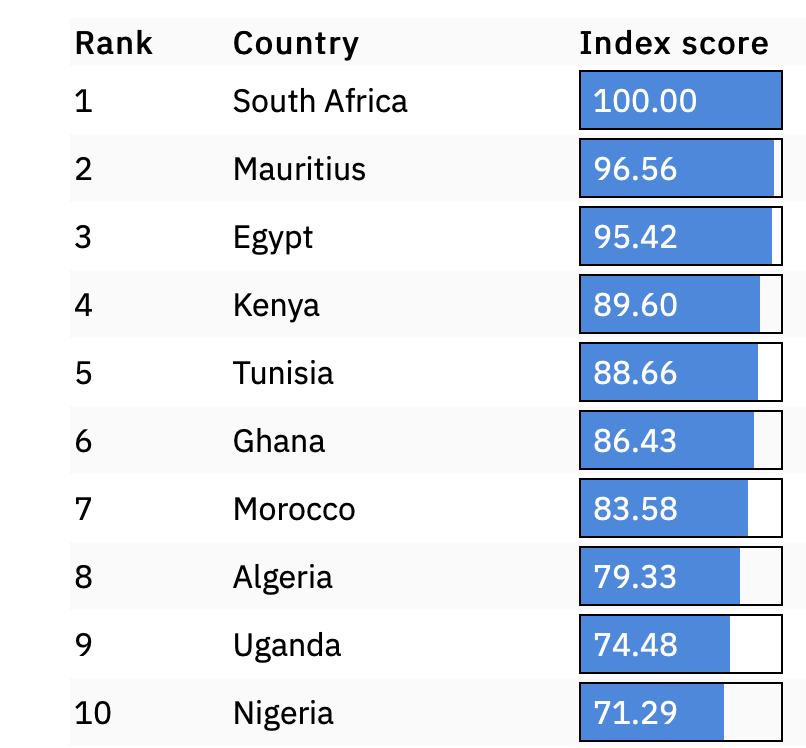
It looks that this first proxy measure is a reasonably good indicator of the present ML-pulse and also ML-readiness we have seen at Deep Learning Indaba. ML research from these countries is the strongest.
Let us look at the number of start-ups in AI/ML in Africa. Which countries do these come from? Again we will recognise the top 10 above: https://startuplist.africa/industry/artificial-intelligence
But what is happening in the other countries which are in the middle or even at the bottom of that list?
To unpack these numbers and translate them in a ML-readiness indicator we need additional data and insights. Here is where you come in! Your insight can help uncover some hidden seeds of the future of ML in Africa.
Tell us what kind of research in ML you do, or know of in your country. Tell us of your experience and challenges.
If you found this post stimulating, please contribute to this discussion by either emailing me at amelia@deeplearningindaba.com or/and also filling in the following survey.
You can email me your thoughts and observations if they do not quite fit into the questions I put in the survey. For example, I recently heard from Mali where Michael Leventhal and his team developed a machine translation tool for Bambara. They say that the integration of their tool in the Google MT platform is still to take place or even to be considered. I think this example points to the fourth dimension in the list above: ‘Process capability and control’. How can smaller players work together with the larger ones? I think an ML-readiness level measure has the potential to help. What do you think?
I look forward to hearing from you. Thank you for your participation.